In today’s digital landscape, online fraud and scams have become increasingly prevalent, making scam analytics a crucial tool for identifying, analyzing, and preventing deceptive activities. With the rise in internet usage, scammers have more opportunities than ever to exploit vulnerabilities. This article dives into the importance of scam analytics, the tools used, and best practices for businesses and individuals looking to protect themselves from fraud.
What is Scam Analytics?
Scam analytics refers to the practice of tracking, analyzing, and understanding fraudulent activities across digital platforms. It combines data collection, machine learning, and predictive analysis to detect patterns and trends in scams. Scam analytics tools analyze large datasets to identify indicators of fraudulent behavior, providing organizations with insights to prevent scams before they occur.
The main objective of scam analytics is to reduce fraud-related losses, protect sensitive data, and enhance the online experience by building a secure environment for users.
How Scam Analytics Works
Scam analytics involves monitoring multiple data sources and using advanced algorithms to detect anomalies. The process generally includes:
- Data Collection: Gathering data from user activity, transaction history, login behavior, and communication patterns.
- Pattern Recognition: Identifying unusual patterns or activities that may indicate fraud, such as rapid login attempts, unusual transaction amounts, or high-risk geographic locations.
- Risk Scoring: Assigning a risk score to each action or transaction, which helps prioritize which actions require further review.
- Alert Generation: Sending real-time alerts when suspicious behavior is detected, allowing for immediate response and intervention.
By focusing on these areas, scam analytics tools can detect threats early and prevent losses. Fraud prevention teams can investigate flagged activities promptly, reducing the chance of successful scams.
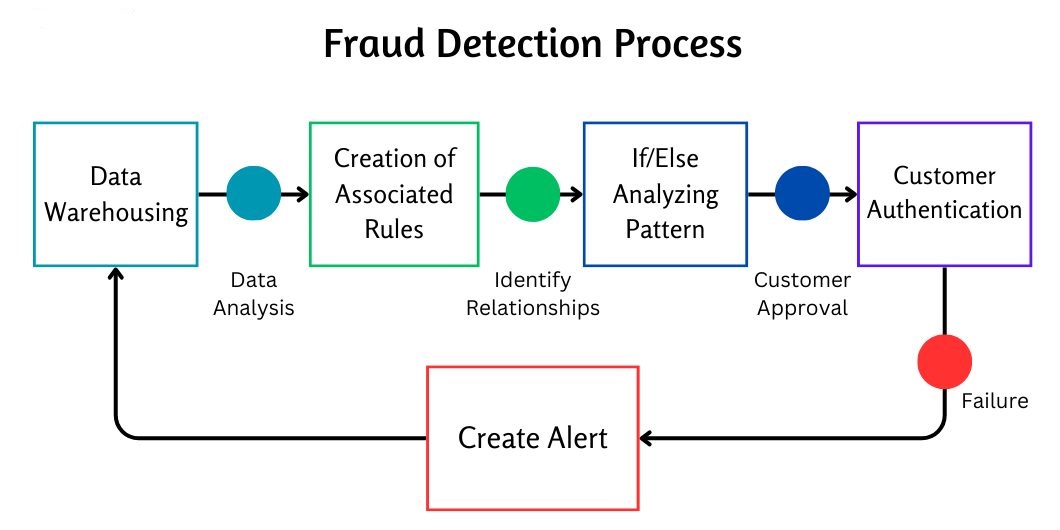
Key Components of Scam Analytics
To achieve effective results, scam analytics integrates several key components:
1. Machine Learning and AI
Machine learning (ML) and artificial intelligence (AI) play pivotal roles in scam analytics. By leveraging AI, organizations can analyze large datasets and identify fraud patterns with increased accuracy. ML models can be trained to recognize both known and emerging scam techniques, making them highly effective in adapting to new threats over time.
The use of supervised and unsupervised learning enables systems to detect not only known scam patterns but also anomalies that may represent new, unknown scams. This adaptability makes machine learning a powerful asset in scam analytics.
2. Predictive Analytics
Predictive analytics uses historical data to anticipate potential fraudulent activity. By studying past scams, predictive analytics tools can identify likely fraud scenarios and generate real-time alerts. Predictive analytics helps organizations proactively address risks, preventing fraud before it escalates.
For example, if a specific account frequently engages in risky behavior, predictive analytics can flag this account for closer monitoring, reducing the likelihood of successful scams.
3. Behavioral Analytics
Behavioral analytics monitors user behavior to detect unusual patterns, such as sudden changes in login times or access from unfamiliar locations. Behavioral analytics can flag actions that deviate from a user’s regular behavior, providing early warnings of possible fraud.
This approach is particularly valuable in distinguishing between legitimate users and scammers, as scammers often behave in ways that differ from regular user patterns. Behavioral analytics, therefore, adds an extra layer of defense in scam detection.
4. Network Analysis
Network analysis focuses on identifying links between accounts, devices, or locations that may indicate organized fraud. This approach is valuable for detecting scams where multiple accounts work together to perpetrate fraudulent activities. By analyzing the connections between different entities, network analysis can uncover hidden relationships that are otherwise difficult to detect.
Network analysis can reveal if several seemingly unrelated accounts are all connected to the same IP address or device, signaling a potential fraud ring.
5. Real-time Monitoring and Alerts
Effective scam analytics tools operate in real-time, enabling immediate response to suspicious activities. Real-time monitoring is essential for businesses, especially in sectors like e-commerce and finance, where delays can result in significant financial losses.
The Importance of Scam Analytics for Businesses
For businesses, scam analytics is not just about protecting data; it’s also about maintaining customer trust and loyalty. Fraud can lead to substantial losses and damage a company’s reputation. By investing in scam analytics, businesses can achieve several benefits:
- Fraud Prevention: Early detection allows companies to prevent fraud before it occurs, safeguarding assets and data.
- Reduced Financial Losses: Scam analytics minimizes the financial impact of scams by preventing fraudulent transactions and activities.
- Improved Customer Experience: By protecting customers from scams, companies enhance the trust and reliability of their platforms.
- Regulatory Compliance: Many industries require stringent data protection measures. Scam analytics helps businesses comply with regulatory standards.
Common Types of Scams and How Scam Analytics Helps Detect Them
Different types of scams require specialized analytics approaches. Here are a few prevalent scams and how scam analytics addresses them:
1. Phishing Scams
Phishing involves tricking users into providing sensitive information, often through fake emails or websites. Scam analytics can detect phishing by analyzing suspicious URLs, monitoring email patterns, and identifying unusual links. Advanced machine learning models can detect the subtle differences between legitimate and phishing attempts, alerting users and security teams in real-time.
2. Account Takeover (ATO)
In Account Takeover (ATO) fraud, attackers gain unauthorized access to user accounts. Scam analytics detects this by monitoring login patterns and unusual activities like multiple failed login attempts, changes in user behavior, or logins from different IP addresses.
3. Payment Fraud
Payment fraud involves unauthorized transactions, often through stolen credit cards or compromised payment details. Scam analytics detects payment fraud by analyzing transaction histories and comparing them to typical user spending behaviors. Any deviation from normal transaction patterns raises an alert, allowing for immediate investigation.
4. Social Engineering Scams
Social engineering scams rely on manipulating individuals to disclose sensitive information. Behavioral analytics in scam analytics can flag unusual communication patterns, helping detect social engineering attempts early.
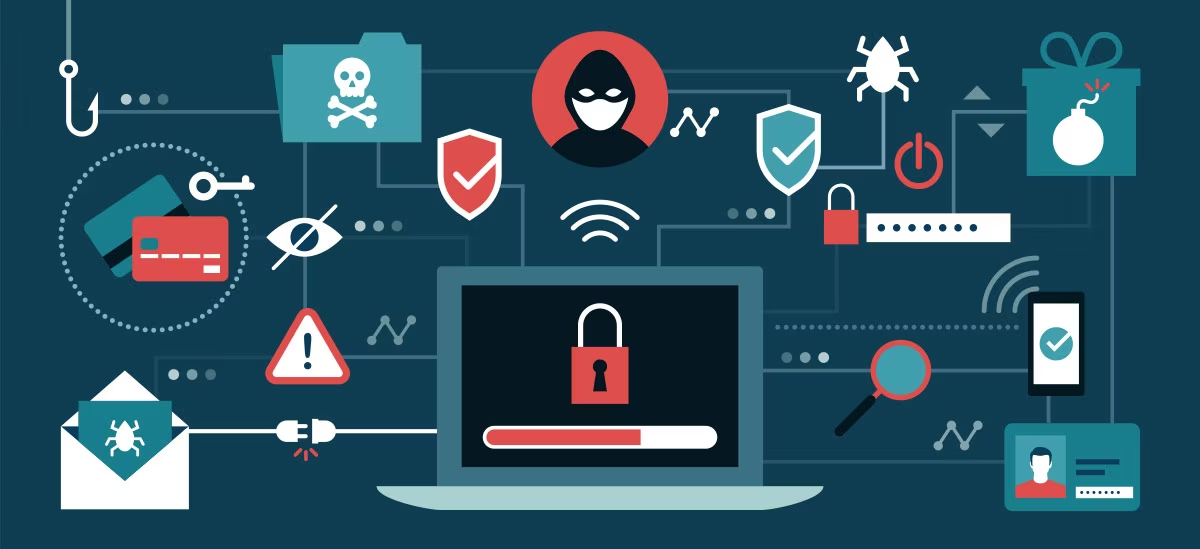
Best Practices in Implementing Scam Analytics
For effective scam analytics, organizations must adopt best practices tailored to their specific needs:
1. Use a Multi-layered Approach
Combining several analytics methods—such as machine learning, predictive analytics, and behavioral analysis—offers a more comprehensive view of potential fraud risks. A multi-layered approach increases the accuracy of detection and reduces false positives.
2. Regularly Update and Train Machine Learning Models
Threat patterns change constantly, so keeping machine learning models updated is essential. Regular training on new scam data ensures that models remain effective in identifying evolving threats.
3. Ensure Data Privacy and Compliance
While scam analytics involves extensive data collection, organizations must handle this data responsibly. Compliance with privacy regulations like GDPR and CCPA is essential to maintain user trust and avoid penalties. Ensure that data collected is stored securely and used only for fraud prevention purposes.
4. Educate Employees and Customers
Scam analytics can be more effective when users are aware of common scams. Training employees and educating customers on recognizing scams, secure login practices, and cautious behavior can improve security across the board.
5. Monitor Performance and Adjust Strategies
Scam analytics should be an ongoing process. Continuously monitor the performance of your analytics tools, adjusting strategies and algorithms based on new data and insights. Regular audits ensure that systems are effective and aligned with the latest threats.
The Future of Scam Analytics
With the rise of sophisticated scams, the future of scam analytics will involve deeper integration with artificial intelligence and advanced automation. As scams become more complex, scam analytics tools will likely evolve to incorporate enhanced capabilities, including:
- Deep learning models to recognize highly complex scam patterns
- Enhanced data sharing across industries to identify scam trends
- Blockchain technology for better transparency and traceability in fraud detection
These developments will help businesses stay one step ahead of cybercriminals and continue to protect valuable assets and customer data.
Conclusion
In a world where scams are constantly evolving, scam analytics is essential for identifying, preventing, and responding to fraud. By leveraging machine learning, predictive analytics, and behavioral analysis, businesses can effectively protect themselves and their customers from financial losses and reputation damage. Implementing scam analytics requires an ongoing commitment to monitoring, adjusting strategies, and educating users, but the benefits far outweigh the costs.
Scam analytics not only provides valuable insights into potential fraud but also plays a crucial role in safeguarding the digital experience. As scams continue to grow in complexity, staying informed and proactive is the best way to prevent losses and maintain customer trust in the digital age.